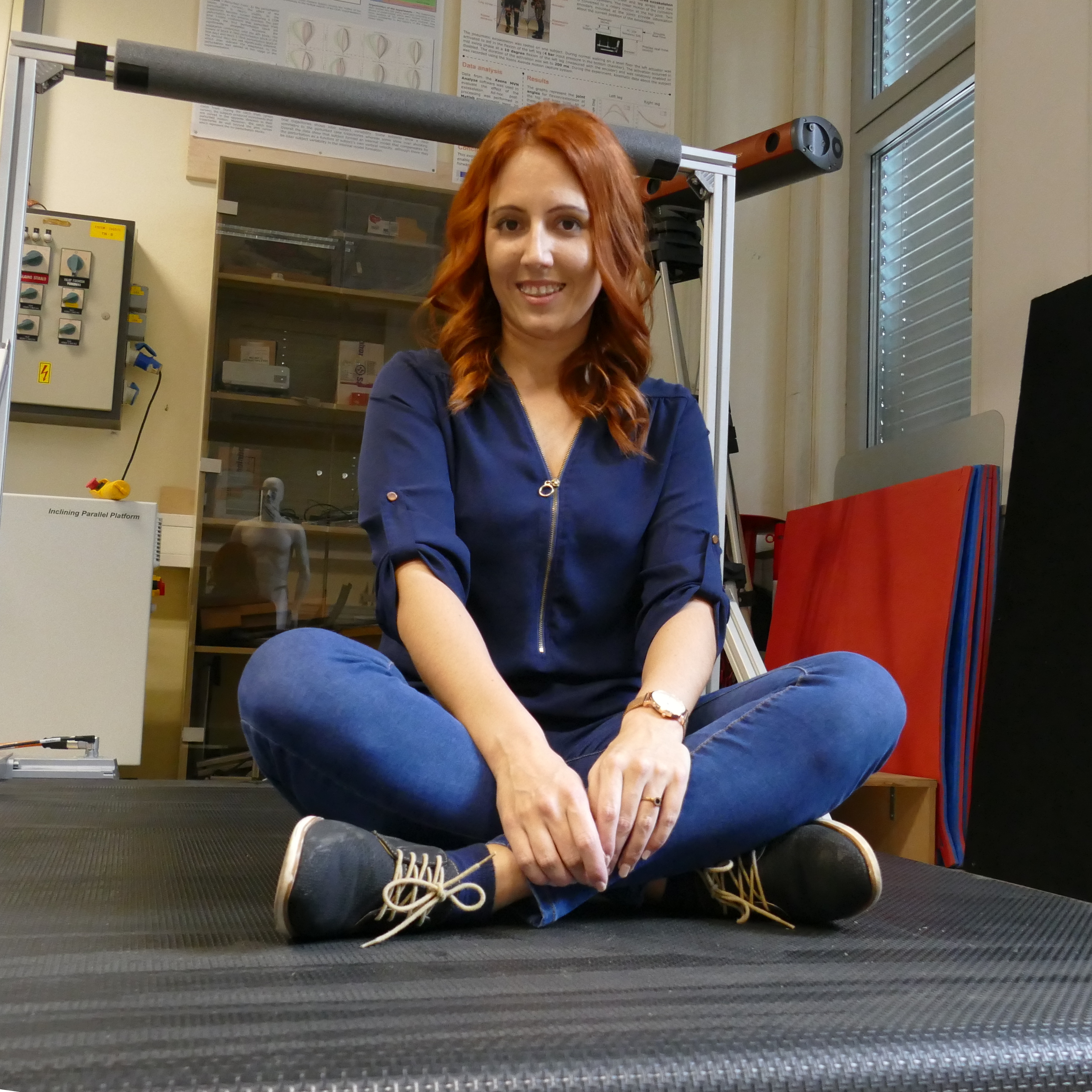
Tjaša Kunavar
PhD Student
Email: tjasa.kunavar@ijs.si
Education
MSc in Computational Neuroscience and Cognitive Robotics, University of Birmingham (2017)
BSc in Biopsychology, University of Primorska (2015)
Research interests
- human whole body motor control
- motor planning
- conscious processes in motor learning
- reinforcement learning
Selected publications
Kunavar, Tjasa; Cheng, Xiaoxiao; Franklin, David W.; Burdet, Etienne; Babič, Jan
Explicit learning based on reward prediction error facilitates agile motor adaptations Journal Article
In: PLOS ONE, vol. 18, iss. 12, pp. e0295274, 2023, ISSN: 1932-6203.
@article{Kunavar2023,
title = {Explicit learning based on reward prediction error facilitates agile motor adaptations},
author = {Tjasa Kunavar and Xiaoxiao Cheng and David W. Franklin and Etienne Burdet and Jan Babi\v{c}},
doi = {10.1371/journal.pone.0295274},
issn = {1932-6203},
year = {2023},
date = {2023-01-01},
urldate = {2023-01-01},
journal = {PLOS ONE},
volume = {18},
issue = {12},
pages = {e0295274},
abstract = {Error based motor learning can be driven by both sensory prediction error and reward prediction error. Learning based on sensory prediction error is termed sensorimotor adaptation, while learning based on reward prediction error is termed reward learning. To investigate the characteristics and differences between sensorimotor adaptation and reward learning, we adapted a visuomotor paradigm where subjects performed arm movements while presented with either the sensory prediction error, signed end-point error, or binary reward. Before each trial, perturbation indicators in the form of visual cues were presented to inform the subjects of the presence and direction of the perturbation. To analyse the interconnection between sensorimotor adaptation and reward learning, we designed a computational model that distinguishes between the two prediction errors. Our results indicate that subjects adapted to novel perturbations irrespective of the type of prediction error they received during learning, and they converged towards the same movement patterns. Sensorimotor adaptations led to a pronounced aftereffect, while adaptation based on reward consequences produced smaller aftereffects suggesting that reward learning does not alter the internal model to the same degree as sensorimotor adaptation. Even though all subjects had learned to counteract two different perturbations separately, only those who relied on explicit learning using reward prediction error could timely adapt to the randomly changing perturbation. The results from the computational model suggest that sensorimotor and reward learning operate through distinct adaptation processes and that only sensorimotor adaptation changes the internal model, whereas reward learning employs explicit strategies that do not result in aftereffects. Additionally, we demonstrate that when humans learn motor tasks, they utilize both learning processes to successfully adapt to the new environments.},
keywords = {},
pubstate = {published},
tppubtype = {article}
}
Kunavar, Tjaša; Jamšek, Marko; Barbiero, Marie; Blohm, Gunnar; Nozaki, Daichi; Papaxanthis, Charalambos; White, Olivier; Babič, Jan
Effects of Local Gravity Compensation on Motor Control During Altered Environmental Gravity Journal Article
In: Frontiers in Neural Circuits, vol. 15, 2021, ISSN: 1662-5110.
@article{Kunavar2021,
title = {Effects of Local Gravity Compensation on Motor Control During Altered Environmental Gravity},
author = {Tja\v{s}a Kunavar and Marko Jam\v{s}ek and Marie Barbiero and Gunnar Blohm and Daichi Nozaki and Charalambos Papaxanthis and Olivier White and Jan Babi\v{c}},
url = {https://www.frontiersin.org/articles/10.3389/fncir.2021.750267/full},
doi = {10.3389/fncir.2021.750267},
issn = {1662-5110},
year = {2021},
date = {2021-10-01},
urldate = {2021-10-01},
journal = {Frontiers in Neural Circuits},
volume = {15},
abstract = {Our sensorimotor control is well adapted to normogravity environment encountered on Earth and any change in gravity significantly disturbs our movement. In order to produce appropriate motor commands for aimed arm movements such as pointing or reaching, environmental changes have to be taken into account. This adaptation is crucial when performing successful movements during microgravity and hypergravity conditions. To mitigate the effects of changing gravitational levels, such as the changed movement duration and decreased accuracy, we explored the possible beneficial effects of gravity compensation on movement. Local gravity compensation was achieved using a motorized robotic device capable of applying precise forces to the subject's wrist that generated a normogravity equivalent torque at the shoulder joint during periods of microgravity and hypergravity. The efficiency of the local gravity compensation was assessed with an experiment in which participants performed a series of pointing movements toward the target on a screen during a parabolic flight. We compared movement duration, accuracy, movement trajectory, and muscle activations of movements during periods of microgravity and hypergravity with conditions when local gravity compensation was provided. The use of local gravity compensation at the arm mitigated the changes in movement duration, accuracy, and muscle activity. Our results suggest that the use of such an assistive device helps with movements during unfamiliar environmental gravity.},
keywords = {},
pubstate = {published},
tppubtype = {article}
}
Arditi, Emir; Kunavar, Tjaša; Ugur, Emre; Babič, Jan; Oztop, Erhan
Inferring Cost Functions Using Reward Parameter Search and Policy Gradient Reinforcement Learning Proceedings Article
In: IECON 2021 – 47th Annual Conference of the IEEE Industrial Electronics Society, pp. 1–6, IEEE, 2021, ISBN: 978-1-6654-3554-3.
@inproceedings{Arditi2021,
title = {Inferring Cost Functions Using Reward Parameter Search and Policy Gradient Reinforcement Learning},
author = {Emir Arditi and Tja\v{s}a Kunavar and Emre Ugur and Jan Babi\v{c} and Erhan Oztop},
url = {https://ieeexplore.ieee.org/document/9589967/},
doi = {10.1109/IECON48115.2021.9589967},
isbn = {978-1-6654-3554-3},
year = {2021},
date = {2021-10-01},
urldate = {2021-10-01},
booktitle = {IECON 2021 \textendash 47th Annual Conference of the IEEE Industrial Electronics Society},
pages = {1--6},
publisher = {IEEE},
abstract = {This study focuses on inferring cost functions of obtained movement data using reward parameter search and pol-icy gradient based Reinforcement Learning (RL). The behavior data for this task is obtained through a series of squat-to-stand movements of human participants under dynamic perturbations. The key parameter searched in the cost function is the weight of total torque used in performing the squat-to-stand action. An approximate model is used to learn squat-to-stand movements via a policy gradient method, namely Proximal Policy Optimization(PPO). A behavioral similarity metric based on Center of Mass(COM) is used to find the most likely weight parameter. The stochasticity in the training result of PPO is dealt with multiple runs, and as a result, a reasonable and a stable Inverse Reinforcement Learning(IRL) algorithm is obtained in terms of performance. The results indicate that for some participants, the reward function parameters of the experts were inferred successfully.},
keywords = {},
pubstate = {published},
tppubtype = {inproceedings}
}
Jamšek, Marko; Kunavar, Tjaša; Bobek, Urban; Rueckert, Elmar; Babič, Jan
Predictive Exoskeleton Control for Arm-Motion Augmentation Based on Probabilistic Movement Primitives Combined With a Flow Controller Journal Article
In: IEEE Robotics and Automation Letters, vol. 6, no. 3, pp. 4417–4424, 2021, ISSN: 2377-3766.
@article{Jamsek2021,
title = {Predictive Exoskeleton Control for Arm-Motion Augmentation Based on Probabilistic Movement Primitives Combined With a Flow Controller},
author = {Marko Jam\v{s}ek and Tja\v{s}a Kunavar and Urban Bobek and Elmar Rueckert and Jan Babi\v{c}},
url = {https://ieeexplore.ieee.org/document/9387088/},
doi = {10.1109/LRA.2021.3068892},
issn = {2377-3766},
year = {2021},
date = {2021-07-01},
urldate = {2021-07-01},
journal = {IEEE Robotics and Automation Letters},
volume = {6},
number = {3},
pages = {4417--4424},
abstract = {There are many work-related repetitive tasks where the application of exoskeletons could significantly reduce the physical effort by assisting the user in moving the arms towards the desired location in space. To make such controlmore user acceptable, the controller should be able to predict the motion of the user and act accordingly. This letter presents an exoskeleton control method that utilizes probabilistic movement primitives to generate predictions of user movements in real-time. These predictions are used in a flow controller, which represents a novel velocity-field-based exoskeleton control approach to provide assistance to the user in a predictive way. We evaluated our approach with a haptic robot, where a group of twelve participants had to perform movements towards different target locations in the frontal plane. We tested whether we could generalize the predictions for new and unknown target locations whilst providing assistance to the user without changing their kinematic parameters. The evaluation showed that we could accurately predict user movement intentions while at the same time significantly decrease the overall physical effort exerted by the participants to achieve the task.},
keywords = {},
pubstate = {published},
tppubtype = {article}
}
Jamšek, Marko; Kunavar, Tjaša; Blohm, Gunnar; Nozaki, Daichi; Papaxanthis, Charalambos; White, Olivier; Babič, Jan
Effects of Simulated Microgravity and Hypergravity Conditions on Arm Movements in Normogravity Journal Article
In: Frontiers in Neural Circuits, vol. 15, pp. 150, 2021, ISSN: 1662-5110.
@article{10.3389/fncir.2021.750176,
title = {Effects of Simulated Microgravity and Hypergravity Conditions on Arm Movements in Normogravity},
author = {Marko Jam\v{s}ek and Tja\v{s}a Kunavar and Gunnar Blohm and Daichi Nozaki and Charalambos Papaxanthis and Olivier White and Jan Babi\v{c}},
url = {https://www.frontiersin.org/article/10.3389/fncir.2021.750176},
doi = {10.3389/fncir.2021.750176},
issn = {1662-5110},
year = {2021},
date = {2021-01-01},
urldate = {2021-01-01},
journal = {Frontiers in Neural Circuits},
volume = {15},
pages = {150},
abstract = {The human sensorimotor control has evolved in the Earth's environment where all movement is influenced by the gravitational force. Changes in this environmental force can severely impact the performance of arm movements which can be detrimental in completing certain tasks such as piloting or controlling complex vehicles. For this reason, subjects that are required to perform such tasks undergo extensive training procedures in order to minimize the chances of failure. We investigated whether local gravity simulation of altered gravitational conditions on the arm would lead to changes in kinematic parameters comparable to the full-body experience of microgravity and hypergravity onboard a parabolic flight. To see if this would be a feasible approach for on-ground training of arm reaching movements in altered gravity conditions we developed a robotic device that was able to apply forces at the wrist in order to simulate micro- or hypergravity conditions for the arm while subjects performed pointing movements on a touch screen. We analyzed and compared the results of several kinematic parameters along with muscle activity using this system with data of the same subjects being fully exposed to microgravity and hypergravity conditions on a parabolic flight. Both in our simulation and in-flight, we observed a significant increase in movement durations in microgravity conditions and increased velocities in hypergravity for upward movements. Additionally, we noted a reduced accuracy of pointing both in-flight and in our simulation. These promising results suggest, that locally simulated altered gravity can elicit similar changes in some movement characteristics for arm reaching movements. This could potentially be exploited as a means of developing devices such as exoskeletons to aid in training individuals prior to undertaking tasks in changed gravitational conditions.},
keywords = {},
pubstate = {published},
tppubtype = {article}
}
Kunavar, Tjaša; Čamernik, Jernej; Kawato, Mitsuo; Oztop, Erhan; Babič, Jan
Failure as a reinforcement in motor learning Proceedings Article
In: Workshop on Mechanism of Brain and Mind 2020, Rusutsu, Japan, 2020.
@inproceedings{Kunavar2020,
title = { Failure as a reinforcement in motor learning},
author = {Tja\v{s}a Kunavar and Jernej \v{C}amernik and Mitsuo Kawato and Erhan Oztop and Jan Babi\v{c}},
year = {2020},
date = {2020-01-04},
booktitle = {Workshop on Mechanism of Brain and Mind 2020},
address = {Rusutsu, Japan},
abstract = {Humans initiate movements and actions to satisfy their needs and secure their survival. It is therefore necessary to understand motor learning in terms of ecological fitness, where behaviour that produces desired result gets reinforced. We investigated whether failure can work as an ecological reinforcement. An experimental paradigm for observing sensorimotor control was used to study human motion in terms of ecological fitness. This type of approach allowed us to take into account the risk of injury as a reinforcement mechanism. Series of squat to stand motions were performed by participants. Backward perturbation to the centre of mass (COM) was applied by a waist pulling mechanism. Participants managed to gradually adapt to perturbation. When perturbation was present, there was a displacement in the anterior direction, caused by adaptation to perturbation. Motor behaviour was adapted following a failed outcome, while the motor behaviour that produced a successful result was retained.},
keywords = {},
pubstate = {published},
tppubtype = {inproceedings}
}
Rottmann, Nils; Kunavar, Tjaša; Babič, Jan; Peters, Jan; Rueckert, Elmar
Learning Hierarchical Acquisition Functions for Bayesian Optimization Proceedings Article
In: 2020 IEEE/RSJ International Conference on Intelligent Robots and Systems (IROS), pp. 5490–5496, 2020.
@inproceedings{9341335,
title = {Learning Hierarchical Acquisition Functions for Bayesian Optimization},
author = {Nils Rottmann and Tja\v{s}a Kunavar and Jan Babi\v{c} and Jan Peters and Elmar Rueckert},
doi = {10.1109/IROS45743.2020.9341335},
year = {2020},
date = {2020-01-01},
urldate = {2020-01-01},
booktitle = {2020 IEEE/RSJ International Conference on Intelligent Robots and Systems (IROS)},
pages = {5490--5496},
abstract = {Learning control policies in robotic tasks requires a large number of interactions due to small learning rates, bounds on the updates or unknown constraints. In contrast humans can infer protective and safe solutions after a single failure or unexpected observation. In order to reach similar performance, we developed a hierarchical Bayesian optimization algorithm that replicates the cognitive inference and memorization process for avoiding failures in motor control tasks. A Gaussian Process implements the modeling and the sampling of the acquisition function. This enables rapid learning with large learning rates while a mental replay phase ensures that policy regions that led to failures are inhibited during the sampling process. The features of the hierarchical Bayesian optimization method are evaluated in a simulated and physiological humanoid postural balancing task. The method out- performs standard optimization techniques, such as Bayesian Optimization, in the number of interactions to solve the task, in the computational demands and in the frequency of observed failures. Further, we show that our method performs similar to humans for learning the postural balancing task by comparing our simulation results with real human data.},
keywords = {},
pubstate = {published},
tppubtype = {inproceedings}
}
Kunavar, Tjaša; Čamernik, Jernej; Oztop, Erhan; Babič, Jan
Characteristics of human whole body motor learning Proceedings Article
In: Turkey Robotics Conference, TORK 2019, Özyegin University, Istanbul, 2019.
@inproceedings{Kunavar2019b,
title = { Characteristics of human whole body motor learning},
author = {Tja\v{s}a Kunavar and Jernej \v{C}amernik and Erhan Oztop and Jan Babi\v{c}},
year = {2019},
date = {2019-06-01},
booktitle = {Turkey Robotics Conference, TORK 2019},
address = {\"{O}zyegin University, Istanbul},
abstract = {Human whole body movement was observed in order to better understand how humans are able to learn motion and adapt to perturbation. This paper presents preliminary results of our experiment and a simple dynamic model that can simulate motion trajectories.},
keywords = {},
pubstate = {published},
tppubtype = {inproceedings}
}
Kunavar, Tjaša; Čamernik, Jernej; Babič, Jan; Oztop, Erhan; Kawato, Mitsuo
Does danger of injury influence human motor adaptation? Proceedings Article
In: Workshop on Mechanism of Brain and Mind 2019, Rusutsu, Japan, 2019.
@inproceedings{Kunavar2019a,
title = { Does danger of injury influence human motor adaptation?},
author = {Tja\v{s}a Kunavar and Jernej \v{C}amernik and Jan Babi\v{c} and Erhan Oztop and Mitsuo Kawato},
year = {2019},
date = {2019-01-06},
booktitle = {Workshop on Mechanism of Brain and Mind 2019},
address = {Rusutsu, Japan},
abstract = {Experimental paradigm for observing sensorimotor control was used to study human whole body motion. It made a step forward from arm-reaching studies to studying movements of the whole human body. Twenty male participants performed a series of squat to stand motions. Following a baseline block, backward perturbation to the centre of mass (COM) was applied by a pulling mechanism. Participants' aim was to successfully stand up. To assess adaptation of motion, the effect of perturbation was quantified using the trajectory area at every trial. Participants managed to gradually adapt to perturbation and significantly decrease the occurrence of failed trials by increasing their trajectory area. Preliminary results show that safety is an important part of motor adaptation process.},
keywords = {},
pubstate = {published},
tppubtype = {inproceedings}
}