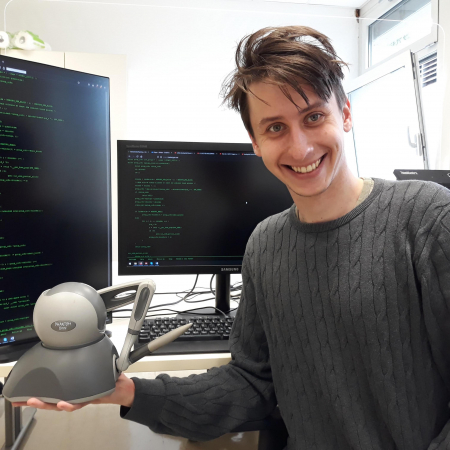
Benjamin Fele
PhD Student
Email: benjamin.fele@ijs.si
Education
MSc in Cognitive Science, University of Ljubljana (2020)
BSc in Computer and Information Science, University of Ljubljana (2017)
Research interests
- reinforcement learning
- meta-learning
- cognitive modeling
- developmental robotics
- generative modeling
Selected Publications
Fele, Benjamin; Lampe, Ajda; Peer, Peter; Štruc, Vitomir
C-VTON: Context-Driven Image-Based Virtual Try-On Network Proceedings Article
In: Proceedings of the IEEE/CVF Winter Conference on Applications of Computer Vision (WACV), 2022.
@inproceedings{fele2022cvton,
title = {C-VTON: Context-Driven Image-Based Virtual Try-On Network},
author = {Benjamin Fele and Ajda Lampe and Peter Peer and Vitomir \v{S}truc},
year = {2022},
date = {2022-01-01},
urldate = {2022-01-01},
booktitle = {Proceedings of the IEEE/CVF Winter Conference on Applications of Computer Vision (WACV)},
abstract = {Image-based virtual try-on techniques have shown great promise for enhancing the user-experience and improving customer satisfaction on fashion-oriented e-commerce platforms. However, existing techniques are currently still limited in the quality of the try-on results they are able to produce from input images of diverse characteristics. In this work, we propose a Context-Driven Virtual Try-On Network (C-VTON) that addresses these limitations and convincingly transfers selected clothing items to the target subjects even under challenging pose configurations and in the presence of self-occlusions. At the core of the C-VTON pipeline are: (i) a geometric matching procedure that efficiently aligns the target clothing with the pose of the person in the input images, and (ii) a powerful image generator that utilizes various types of contextual information when synthesizing the final try-on result. C-VTON is evaluated in rigorous experiments on the VITON and MPV datasets and in comparison to state-of-the-art techniques from the literature. Experimental results show that the proposed approach is able to produce photo-realistic and visually convincing results and significantly improves on the existing state-of-the-art.},
keywords = {},
pubstate = {published},
tppubtype = {inproceedings}
}
Fele, Benjamin; Babič, Jan; Pollak, Senja; Žnidaršič, Martin
Evaluation of curriculum learning algorithms using computational creativity inspired metrics Proceedings Article
In: Hedblom, Maria M. (Ed.): Proceedings of the Thirteenth International Conference on Computational Creativity, ICCC'22, pp. 364–373, Bolzano, Italy, 2022.
@inproceedings{Fele2022,
title = {Evaluation of curriculum learning algorithms using computational creativity inspired metrics},
author = {Benjamin Fele and Jan Babi\v{c} and Senja Pollak and Martin \v{Z}nidar\v{s}i\v{c}},
editor = {Maria M. Hedblom},
url = {https://computationalcreativity.net/iccc22/wp-content/uploads/2022/06/ICCC-2022_5L_Fele-et-al..pdf},
year = {2022},
date = {2022-01-01},
urldate = {2022-01-01},
booktitle = {Proceedings of the Thirteenth International Conference on Computational Creativity, ICCC'22},
pages = {364--373},
address = {Bolzano, Italy},
abstract = {Curriculum learning, especially in robotics, is an active research field aiming to devise algorithms that speed up knowledge acquisition by proposing sequences of tasks an agent should train on. We focus on curriculum generation in reinforcement learning, where various methods are currently compared based on the agent's performance in terms of rewards on a predefined distribution of target tasks. We want to extend this singular characterization of existing algorithms by introducing metrics inspired by notions from the field of computational creativity. Namely, we introduce surprise, novelty, interestingness, and typicality that quantify various aspects of tasks stochastically proposed by the curriculum learning algorithms for the learner to train on. We model proposed tasks with Gaussian mixture models which enable their probabilistic interpretation, and use Hellinger distances between distributions and training rewards in formulation of the proposed metrics. Results are presented for eight curriculum learning algorithms showcasing differences in prioritization of various aspects of task creation and statistically different mean metric values when comparing agent's best and worst training runs. The latter finding is not only useful for analysis of existing algorithms, but potentially also provides guidance for design of future curriculum learning methods.},
keywords = {},
pubstate = {published},
tppubtype = {inproceedings}
}
Fele, Benjamin; Takác, Martin
A connectionist model of acquisition of noun phrases with syntactic bootstrapping Proceedings Article
In: Kognícia a umelý život 2019, pp. 31-32, 2019, ISBN: 978-80-223-4720-4.
@inproceedings{fele2019connectionist,
title = {A connectionist model of acquisition of noun phrases with syntactic bootstrapping},
author = {Benjamin Fele and Martin Tak\'{a}c},
url = {http://cogsci.fmph.uniba.sk/kuz2019/files/zbornik/fele-takac.pdf},
isbn = {978-80-223-4720-4},
year = {2019},
date = {2019-01-01},
urldate = {2019-01-01},
booktitle = {Kogn\'{i}cia a umel\'{y} \v{z}ivot 2019},
pages = {31-32},
abstract = {We present a model of acquisition of noun phrases as descriptions of objects in a grounded setting. The model uses cross-situational learning to acquire mappings between words and sensory properties represented in self-organising maps. We also explore syntactic bootstrapping\textemdashusing the syntactic knowledge to help meaning acquisition and vice versa. The model can reliably reconstruct inputs and is applicable in real-world scenarios.},
keywords = {},
pubstate = {published},
tppubtype = {inproceedings}
}
Fele, Benjamin
Dynamic Determination of Appropriate Training Instances Using Reinforcement Learning Proceedings Article
In: MEi: CogSci Conference 2019, pp. 23, 2019, ISBN: 978-80-223-4740-2.
@inproceedings{fele2019dynamic,
title = {Dynamic Determination of Appropriate Training Instances Using Reinforcement Learning},
author = {Benjamin Fele},
url = {https://www.meicogsci.eu/downloads/MEiCogSci_Conference_Proceedings_2019.pdf},
isbn = {978-80-223-4740-2},
year = {2019},
date = {2019-01-01},
urldate = {2019-01-01},
booktitle = {MEi: CogSci Conference 2019},
pages = {23},
keywords = {},
pubstate = {published},
tppubtype = {inproceedings}
}
Luštrek, Mitja; Fele, Benjamin; Reščič, Nina; Janko, Vito
Counting bites with a smart watch Proceedings Article
In: Slovenian Conference on Artificial Intelligence: proceedings of the 22nd International Multiconference Information Society-IS, pp. 49–52, 2019.
@inproceedings{luvstrek2019counting,
title = {Counting bites with a smart watch},
author = {Mitja Lu\v{s}trek and Benjamin Fele and Nina Re\v{s}\v{c}i\v{c} and Vito Janko},
url = {http://ai.ijs.si/MitjaL/documents/Counting_bites_with_a_smart_watch-IS-19.pdf},
year = {2019},
date = {2019-01-01},
urldate = {2019-01-01},
booktitle = {Slovenian Conference on Artificial Intelligence: proceedings of the 22nd International Multiconference Information Society-IS},
pages = {49--52},
abstract = {The work described in this paper is a part of the WellCo project, which is developing a virtual coach for healthy lifestyle. An important aspect of a healthy lifestyle is nutrition, and knowing as much as possible about the users’ current nutrition can contribute to better coaching. We therefore set out to count the number of times the users take food to their mouths (bites) using smartwatch sensors. This enables identifying the meals as well as estimating the caloric intake and the speed of eating. We compare three approaches: two that rely on classical machine learning and hidden Markov models, and one that uses deep learning. The F-scores of the approaches range from 0.63 to 0.91, and the percentages of miscounted bites from 6.9 % to 10.7 %, with a different approach scoring best on each metric.},
keywords = {},
pubstate = {published},
tppubtype = {inproceedings}
}